[python 데이터 분석 실습] 코로나 19 2021 현재 시점 데이터 동적 시각화 분석하기 2편
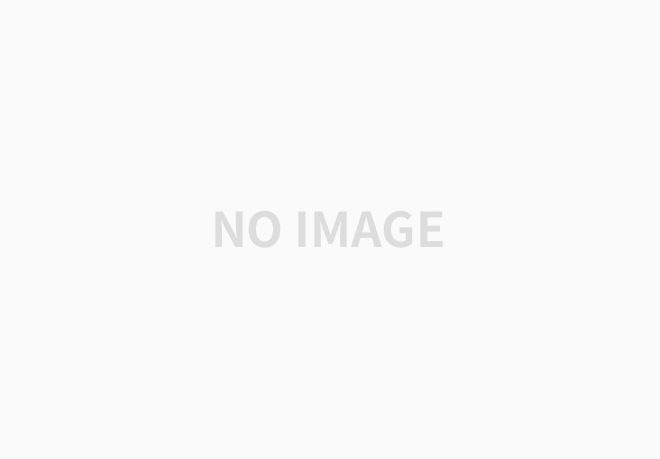
파이썬 데이터 분석 코로나19 데이터 분석 실습 두번째 시간 입니다.
1편을 올리고 시간이 좀 늦었습니다.
이번편에서는 남은 전처리를 좀 하고, 시각화 하는것을 실습 하겠습니다.
바로 그럼 시작 하도록 하겠습니다.
<지난편 보러가기>
2021.03.29 - [Data Science] - [python 데이터 분석 실습] 코로나 19 2021 현재 시점 분석하기 1편
[python 데이터 분석 실습] 코로나 19 2021 현재 시점 분석하기 1편
코로나 19 2021 현재 시점 python으로 데이터 분석하기 안녕하세요. 파이썬 데이터 분석 실습 쉽게 따라해보기~ 이번 시간은 코로나 19의 2021년 현재 상황 분석 하기 입니다. 우리 세상을 뒤덮고, 일
stricky.tistory.com
데이터 전처리
저번시간에 데이터 전처리가 다 된줄 알았는데 좀 더 있더라구요.
이어서 가도록 하겠습니다.
이전 시간에 데이터를 크게, 미국와 그 외 전세계로 나누었는데, 이번에는 ww_df 라는 데이터 프레임을 만들어서 그 안에 전세계 데이터의 new_case와 growth factor 값을 날짜별로 구해 보도록 하겠습니다.
ww_df = train.groupby('date')[['confirmed', 'fatalities']].sum().reset_index()
ww_df['new_case'] = ww_df['confirmed'] - ww_df['confirmed'].shift(1)
ww_df['growth_factor'] = ww_df['new_case'] / ww_df['new_case'].shift(1)
ww_df.tail()
아래와 같이 데이터가 완성이 됩니다.
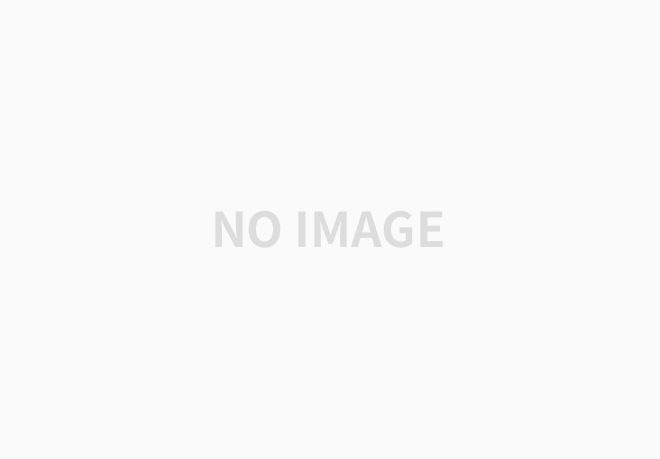
그리고 다음 스텝은 날짜별로 진단, 확진, 사망등의 통계를 내기 위해 아래와 같이 누적수치를 구합니다.
ww_melt_df = pd.melt(ww_df, id_vars=['date'], value_vars=['confirmed', 'fatalities', 'new_case'])
ww_melt_df
아래와 같은 결과가 나오게 됩니다.
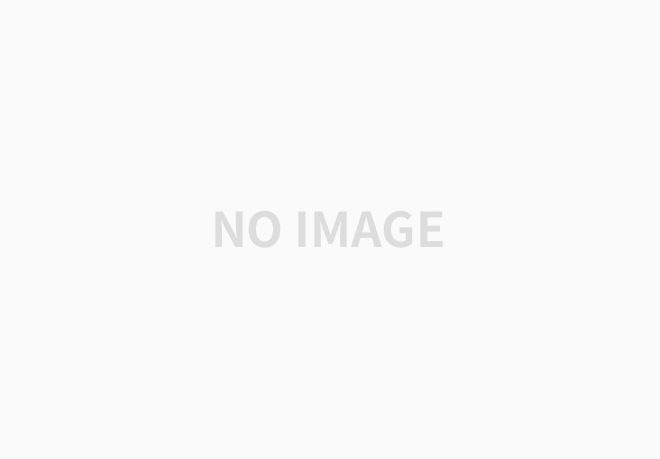
데이터 시각화
데이터 시각화를 하기 앞서서 몇가지 패키지를 import 해주겠습니다.
# --- plotly ---
from plotly import tools, subplots
import plotly.offline as py
py.init_notebook_mode(connected=True)
import plotly.graph_objs as go
import plotly.express as px
import plotly.figure_factory as ff
import plotly.io as pio
pio.templates.default = "plotly_dark"
import matplotlib.pyplot as plt
import seaborn as sns
그럼 방금 위에서 만들었던 데이터셋이 있죠. ww_melt_df 를 이용해서 그래프를 그려보겠습니다.
fig = px.line(ww_melt_df, x="date", y="value", color='variable',
title="Worldwide Confirmed/Death Cases Over Time")
fig.show()
아래와 같이 확대도 할 수 있고, 데이터를 잘 살펴볼수 있는 좋은 그래프가 생성 되는것을 확인 할 수 있습니다.
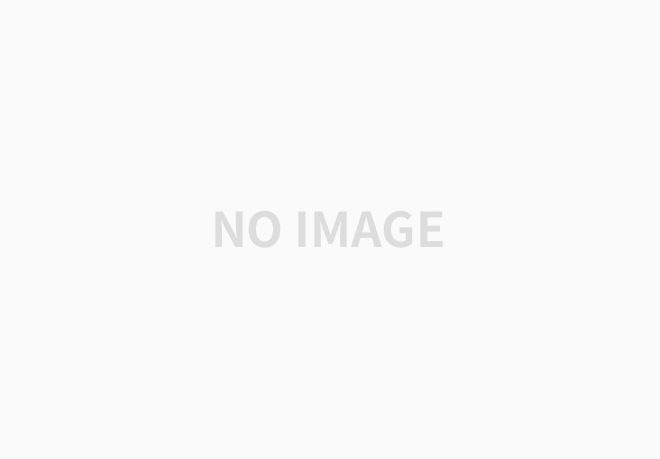
다른 방식으로 그려보겠습니다. Log scale을 이용했습니다.
fig = px.line(ww_melt_df, x="date", y="value", color='variable',
title="Worldwide Confirmed/Death Cases Over Time (Log scale)",
log_y=True)
fig.show()
아래와 같이 좀 더 눈에 들어오는 차트를 볼 수 있습니다.
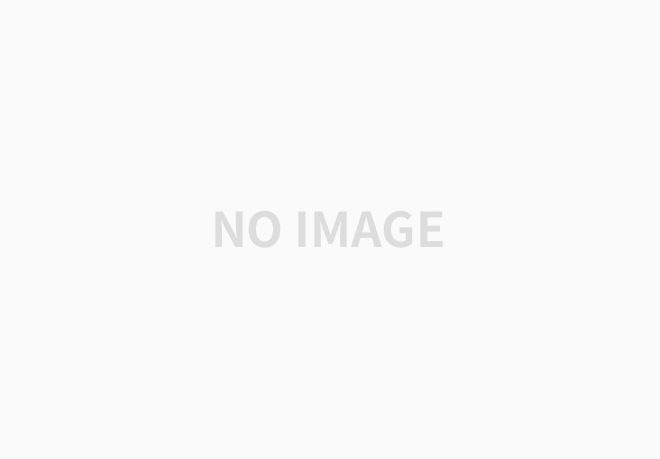
다음은 사망률을 가지고 그래프를 그려보겠습니다.
아래와 같이 코드를 작성 합니다.
ww_df['mortality'] = ww_df['fatalities'] / ww_df['confirmed']
fig = px.line(ww_df, x="date", y="mortality",
title="Worldwide Mortality Rate Over Time")
fig.show()
차트를 그려봅니다.
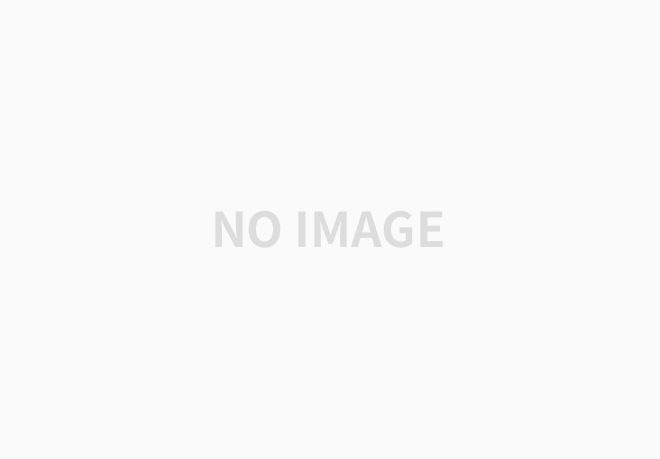
아무래도 초기에 사망률이 높아졌다가 점점 안정을 찾는 모습을 확인 할 수 있습니다.
경험치의 차이겠죠.
다음은 Growth Factor 차트를 그려보도록 하겠습니다.
fig = px.line(ww_df, x="date", y="growth_factor",
title="Worldwide Growth Factor Over Time")
fig.add_trace(go.Scatter(x=[ww_df['date'].min(), ww_df['date'].max()], y=[1., 1.], name='Growth factor=1.', line=dict(dash='dash', color=('rgb(255, 0, 0)'))))
fig.update_yaxes(range=[0., 5.])
fig.show()
차트는 아래와 같이 보여집니다.
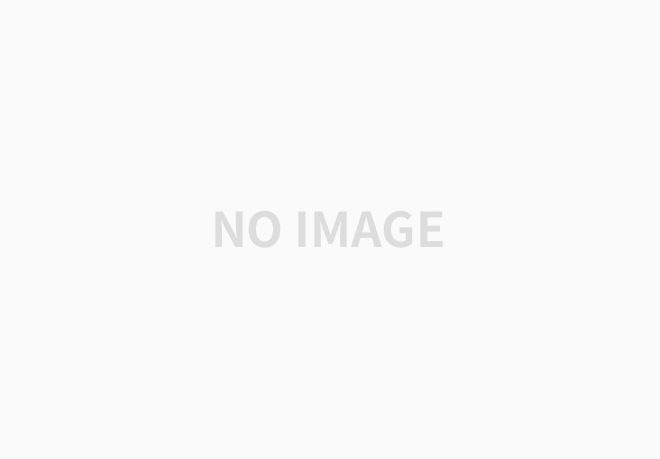
확실히 처음 유행이 쭉퍼지다가 2021년 1월 직전에 또 한번의 유행이 왔음을 Growth Fator를 통해 확인 할 수 있습니다.
국가별 분석
다음은 국가별로 코로나19의 추이가 어떻게 되는지를 확인 해 보겠습니다.
우선 국가별 사망자와 진단 데이터를 생성 합니다.
country_df = train.groupby(['date', 'country'])[['confirmed', 'fatalities']].sum().reset_index()
country_df.tail()
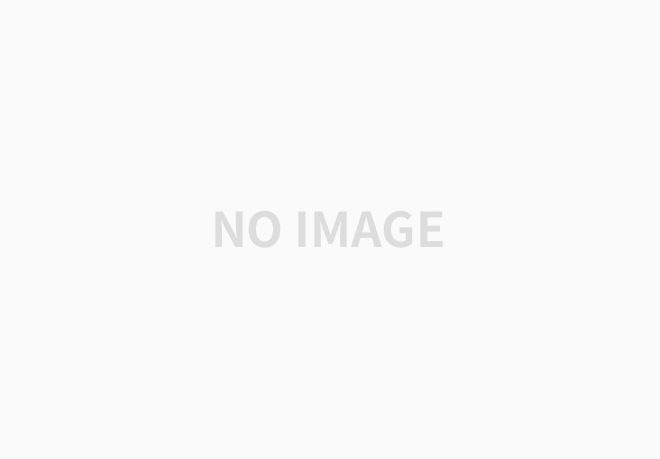
유니크한 국가명을 따로 저장 하겠습니다.
countries = country_df['country'].unique()
print(f'{len(countries)} countries are in dataset:\n{countries}')
총 192개의 국가가 있네요.
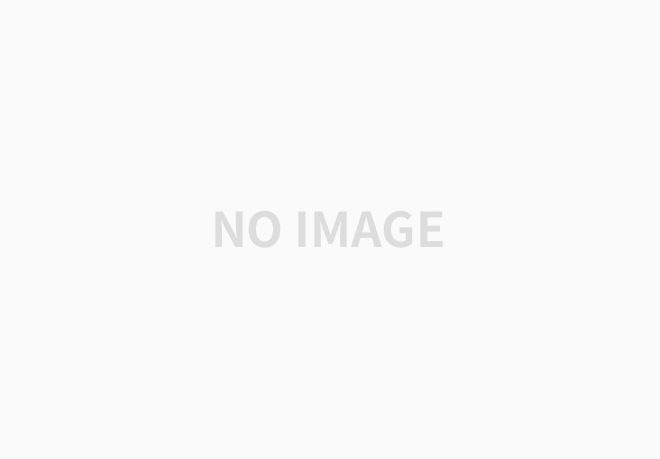
그리고, 각 국가들이 얼마나 많은 진단을 하고 있는지를 확인 해보겠습니다.
confirmed 데이터를 활용 합니다.
target_date = country_df['date'].max()
print('Date: ', target_date)
for i in [1, 100, 10000, 100000, 1000000, 10000000]:
n_countries = len(country_df.query('(date == @target_date) & confirmed > @i'))
print(f'{n_countries} countries have more than {i} confirmed cases')
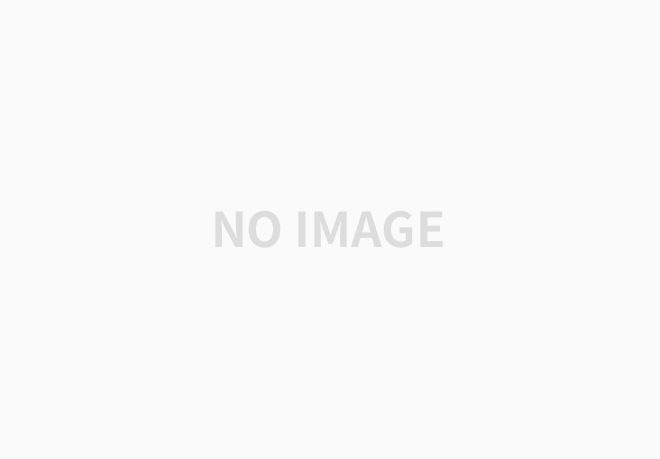
이번에는 confirmed 값이 1000 이상인 국가들을 가지고 탑 다운 차트를 그려보도록 하겠습니다.
아래와 같이 코드를 작성 합니다.
top_country_df = country_df.query('(date == @target_date) & (confirmed > 1000)').sort_values('confirmed', ascending=False)
top_country_melt_df = pd.melt(top_country_df, id_vars='country', value_vars=['confirmed', 'fatalities'])
우선 이렇게 데이터를 만들고 다음 코드로 차트를 그려봅니다.
fig = px.bar(top_country_melt_df.iloc[::-1],
x='value', y='country', color='variable', barmode='group',
title=f'Confirmed Cases/Deaths on {target_date}', text='value', height=3000, orientation='h')
fig.show()
우리 대한민국은 너무 아래 있어서 찾기 어렵네요.
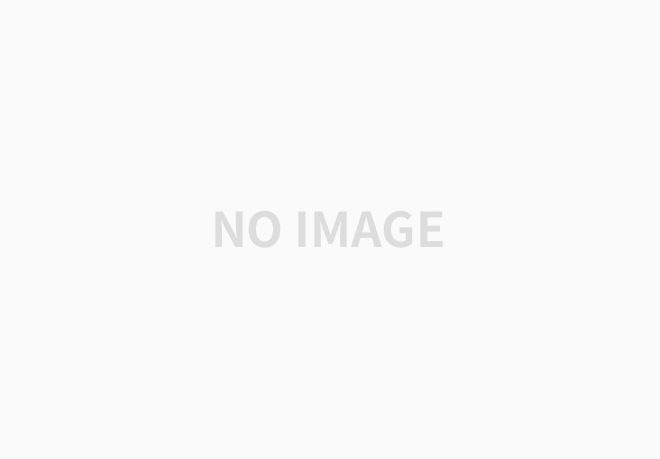
이번엔 Top30 국가들의 진단 데이터를 차트로 그려봅니다.
top30_countries = top_country_df.sort_values('confirmed', ascending=False).iloc[:30]['country'].unique()
top30_countries_df = country_df[country_df['country'].isin(top30_countries)]
fig = px.line(top30_countries_df,
x='date', y='confirmed', color='country',
title=f'Confirmed Cases for top 30 country as of {target_date}')
fig.show()
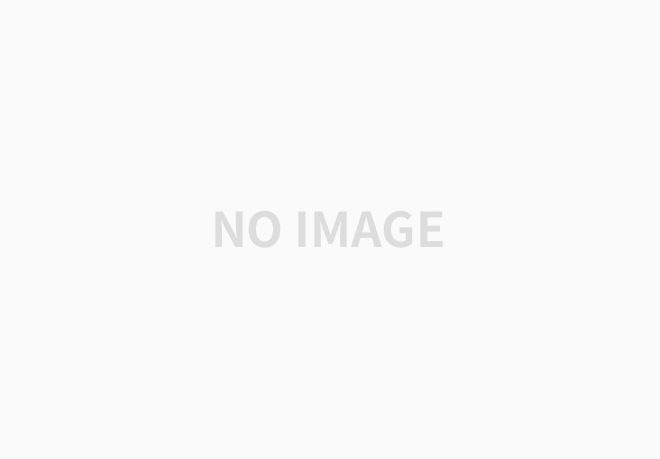
유럽과 남미 국가들이 많이 보이는것 같은건 기분탓이겠죠?
사망자 데이터를 이용해서도 그려봅니다.
top30_countries = top_country_df.sort_values('fatalities', ascending=False).iloc[:30]['country'].unique()
top30_countries_df = country_df[country_df['country'].isin(top30_countries)]
fig = px.line(top30_countries_df,
x='date', y='fatalities', color='country',
title=f'Fatalities for top 30 country as of {target_date}')
fig.show()
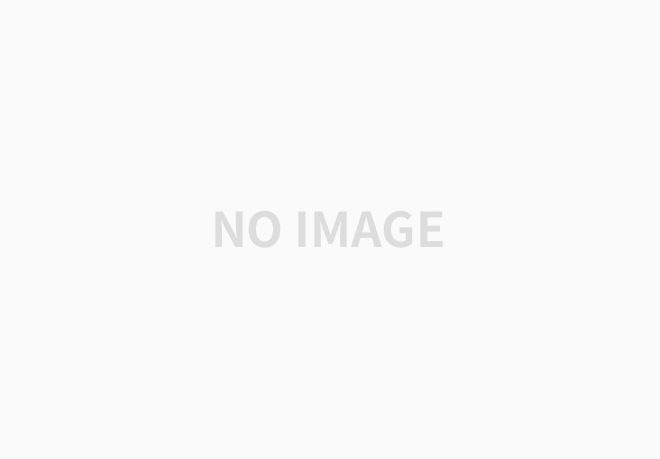
국가 구성은 비슷한것 같습니다.
사망률을 가지고 한번 데이터 시각화를 해봅니다. 그러기 위해서 데이터를 만들어야죠. 아래와 같이 만들어 줍니다.
top_country_df = country_df.query('(date == @target_date) & (confirmed > 100)')
top_country_df['mortality_rate'] = top_country_df['fatalities'] / top_country_df['confirmed']
top_country_df = top_country_df.sort_values('mortality_rate', ascending=False)
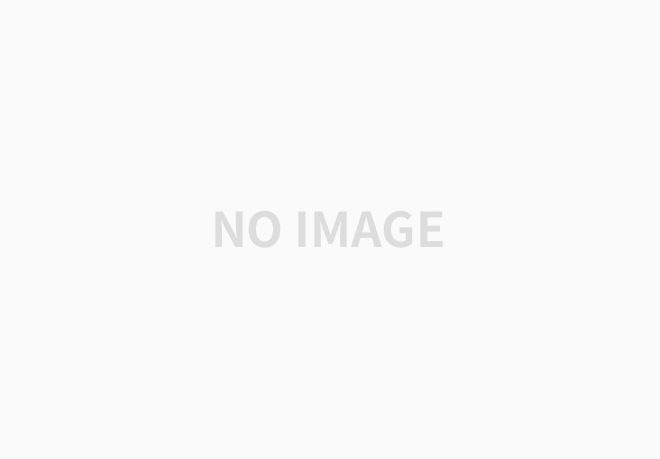
코로나 사망률이서는 예멘이 가장 높은것을 보여줍니다. 아무래도 국가별 확진자수와는 또 별개로 의료수준이나 대응력을 평가 할만한 수치인듯 합니다.
반대로 사망률이 낮은 나라순 입니다.
fig = px.bar(top_country_df[-30:],
x='mortality_rate', y='country',
title=f'Mortality rate LOW: top 30 countries on {target_date}', text='mortality_rate', height=800, orientation='h')
fig.show()
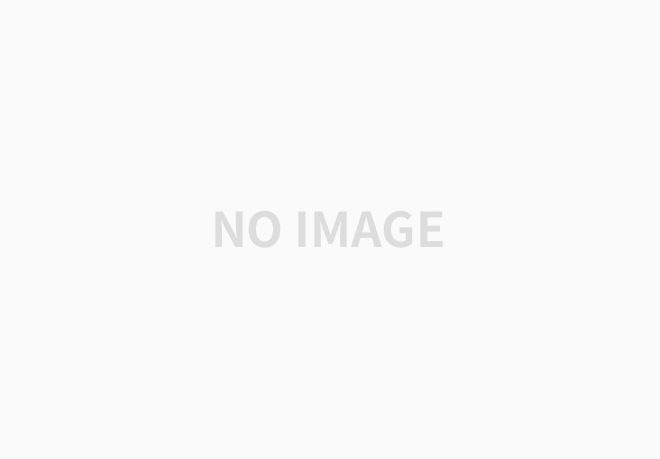
이번에는 세계지도위에 시각화를 해보겠습니다.
진단과 사망률을 가지고 세가지 차트를 만들어 봅니다.
우선 데이터 부터 만들구요.
all_country_df = country_df.query('date == @target_date')
all_country_df['confirmed_log1p'] = np.log10(all_country_df['confirmed'] + 1)
all_country_df['fatalities_log1p'] = np.log10(all_country_df['fatalities'] + 1)
all_country_df['mortality_rate'] = all_country_df['fatalities'] / all_country_df['confirmed']
먼저 진단수 시각화 입니다.
fig = px.choropleth(all_country_df, locations="country",
locationmode='country names', color="confirmed_log1p",
hover_name="country", hover_data=["confirmed", 'fatalities', 'mortality_rate'],
range_color=[all_country_df['confirmed_log1p'].min(), all_country_df['confirmed_log1p'].max()],
color_continuous_scale="peach",
title='Countries with Confirmed Cases')
# I'd like to update colorbar to show raw values, but this does not work somehow...
# Please let me know if you know how to do this!!
trace1 = list(fig.select_traces())[0]
trace1.colorbar = go.choropleth.ColorBar(
tickvals=[0, 1, 2, 3, 4, 5, 6, 7, 8],
ticktext=['1', '10', '100', '1000','10000', '100000', '1000000', '10000000', '100000000'])
fig.show()
차트는 아래와 같이 나옵니다.
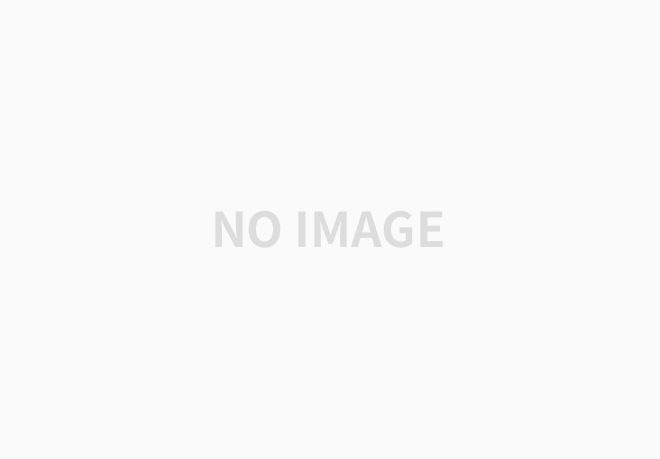
다음은 사망률 데이터 시각화 입니다.
fig = px.choropleth(all_country_df, locations="country",
locationmode='country names', color="mortality_rate",
hover_name="country", range_color=[0, 0.10],
color_continuous_scale="peach",
title='Countries with mortality rate')
fig.show()
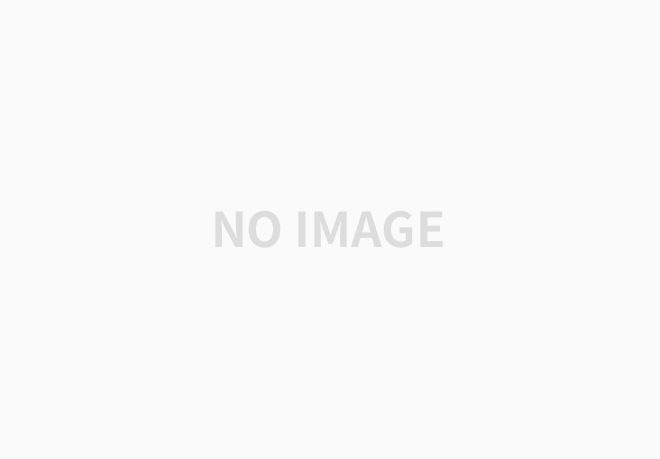
자, 이번 2편은 여기까지로 줄이겠습니다.
다음 3편에서 이어서 진행 하도록 하겠습니다.
코로나 데이터 시각화 조금이라도 도움이 되셨는지 모르겠습니다.
감사합니다.
코로나 조심하시구요!
#다음편 보러가기
2021.05.07 - [Data Science] - [python] 파이썬 데이터 분석 코로나 19 동적 시각화 분석하기 3편
[python] 파이썬 데이터 분석 코로나 19 동적 시각화 분석하기 3편
[python] 파이썬 데이터 분석 코로나 19 동적 시각화 분석하기 3편 코로나 19 데이터를 이용한 동적 시각화 분석 세번째 시간 입니다. 아직 1, 2편을 안보신 분들은 아래 링크로 이동 하셔서 1,
stricky.tistory.com
by.sTricky
'Data Science' 카테고리의 다른 글
구글 빅쿼리(BigQuery) 시작하기 및 datagrip 연동 안내 (0) | 2021.07.27 |
---|---|
[python] 파이썬 데이터 분석 코로나 19 동적 시각화 분석하기 3편 (0) | 2021.05.07 |
[python 데이터 분석 실습] 코로나 19 2021 현재 시점 분석하기 1편 (0) | 2021.03.29 |
캐글 데이터 시각화 넷플릭스(netflix) 데이터를 이용한 데이터 분석 실습 (3) (0) | 2021.03.09 |
캐글 넷플릭스(netflix) 데이터를 이용한 데이터 분석 실습 (2) (0) | 2021.03.03 |